In Marketing BS newsletters, I regularly argue that fancy trends can distract us from the simple fundamentals that matter. Too many of us drop the ball on straightforward tactics like “promptly responding to potential customers on the channel of their choice,” yet we somehow find the time to fantasize about the latest martech gimmick. Many years ago (maybe 2013?), I wrote a blog post about the hype surrounding “Big Data.” I warned marketers that obsessions with Big Data can divert attention from strategies that are not only more impactful, but also much easier to implement. I have shared similar opinions about personalization and machine learning. To be clear: I don’t think these technologies are “bad” per se. Unless you are a well-resourced company like Facebook, Google, or Amazon, your time is better spent on other priorities.
But just because the fancy trends are USUALLY a waste of time doesn’t mean they are ALWAYS a waste of time. If you follow tech news even peripherally, you already know what I am talking about: GPT-3. But if aren’t connected to tech, you probably aren’t familiar with this acronym. After all, I did not see any coverage of GPT-3 in recent issues of the New York Times, Wall Street Journal, Washington Post, USA Today, Guardian, or The Economist.
As someone who is rarely prone to hyperbole, I will go out on a limb and say: “I think GPT-3 is really big.” Most people still cannot access the software, but I will explain a workaround to let you play with the technology. Buckle up!
—Edward
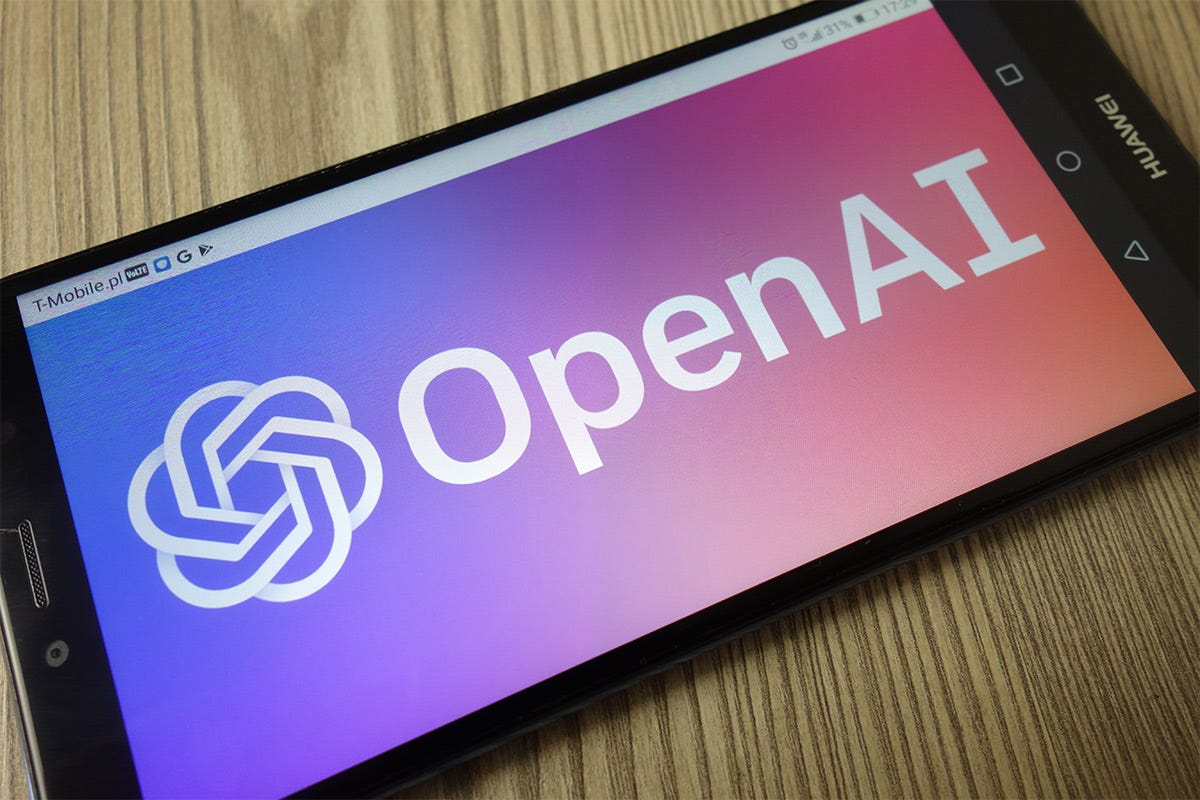
Artificial intelligence
On June 11, OpenAI — a San Francisco-based artificial intelligence lab — announced an API (application programming interface) that would allow a limited number of users to experiment with their new GPT-3 tool. Here is OpenAI’s own description of their work:
Unlike most AI systems which are designed for one use-case, the API today provides a general-purpose “text in, text out” interface, allowing users to try it on virtually any English language task.... Given any text prompt, the API will return a text completion, attempting to match the pattern you gave it. You can “program” it by showing it just a few examples of what you’d like it to do; its success generally varies depending on how complex the task is. The API also allows you to hone performance on specific tasks by training on a dataset (small or large) of examples you provide, or by learning from human feedback provided by users or labelers.
GPT-3 is the third iteration of OpenAI’s “Generative Pre-Trained Transformer.” For a detailed explanation of its basic functions, applications, and limitations, I HIGHLY recommend Leon Lin’s 6000+ word essay (and you thought some issues of Marketing BS were long!). Quite simply, GPT-3 is a “word prediction engine”: it analyzes the input text and produces the output text. I realize that the description alone does not sound particularly impressive. And the first version of GPT (released in 2018) wasn’t that impressive either. But GPT-2 (released in early 2019) WAS impressive. At the time, Scott Alexander of Slatestarcodex wrote about GPT-2, calling it “a step towards general intelligence.” He contended that the human brain is essentially a “prediction engine.” We spend our lives trying to predict what will happen next, based on events that have already happened. Alexander goes so far as to compare the writing of GPT-2 to the way our brains work when we dream — both can generate predictive content, just not very well. Indeed, many of GPT-2’s writing experiments conveyed the feeling of dreams: things start down one path before distractions and tangents disrupt the sense of coherence. (At least my dreams are usually like that.)
GPT-2 was “trained” with 1.5 billion “parameters” (pieces of data). The program analyzes the inputted content and then builds a probability distribution for which words would logically appear next. Next, GPT-2 uses a randomization function (the standard deviation of which can be shifted up or down) to choose a word. And then it repeats the process for the next word, and so on. The process is not lightning fast, but it’s not slow, either — it takes a few seconds to produce a sentence or two.
GPT-3 uses an almost identical model, but OpenAI increased the parameters by two orders of magnitude — 175 billion pieces of data, which is practically the entire internet. The results are shocking. While GPT-2 seemed like an entertaining game, GPT-3 looks like a revolutionary game-changer. Right out of the box, GPT-3 seems capable of just about anything.
Early experiments
Let’s tour some of the examples from the (awestruck) people who received early access to GPT-3.
Sharif Shameem might have set off the GPT-3 frenzy with a short video he posted on Twitter. Shameem provided the tool with two examples of (1) a description of what he wanted, and (2) the JSX code to build that type of element. As a test, Shameem requested: “a button that looks like a watermelon.” GPT-3 responded with both an image and the code.
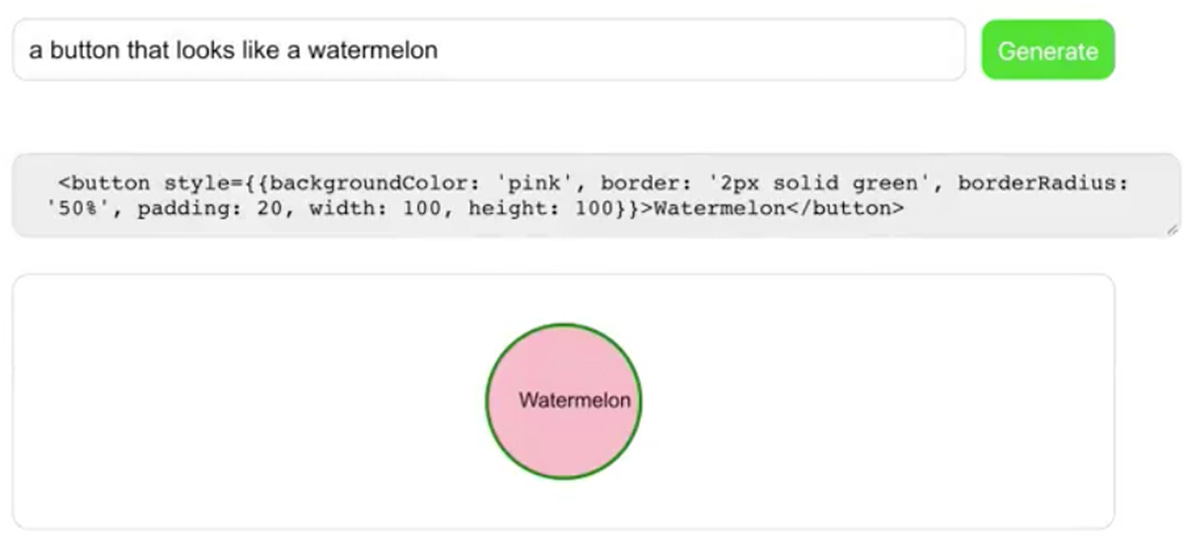
Remember: GPT-3’s only domain-specific “training” was a pair of samples provided by Shameem.
Amjad Masad took the opposite approach. He supplied GPT-3 with a piece of code and then asked for a description of its function using everyday English. GPT-3 offered a clear and accurate explanation; in further tests, the software even gave suggestions to improve the code.
Unlike other AI platforms, GPT-3’s language tools are not limited to narrow contexts like coding. Users can leverage the tool for literary tasks.
For example, Andrew Mayne tested its ability to generate text at different comprehension levels. He inputted the following text: “My second grader asked me what this passage means: [content from wikipedia on quantum computing]. I rephrased it for him in plain language second grader can understand.” Here is the output from GPT-3:
Some people think that if you had one hundred people working on one problem for one hundred years you could not figure out how to solve it with a regular computer but if you had one hundred people working on one problem for one hundred years using a special kind of computer called a Quantum Computer you could figure out how to solve it.
Mayne repeated the experiment by demanding an explanation for an eighth grader:
When we say quantum computing, we mean computing based on quantum physics. With classical computing we perform calculations one bit at a time. With quantum computing we try to take advantage of a superposition between 1 and 0. This is what we mean by qubits. This allows us to make connections between pairs of bits (qubits) using quantum logic gates. Quantum computing allows us to perform computations faster than classical computing.
Imagine the limitless possibilities for a tool that can take any piece of text and adjust its level of complexity for different levels of reading comprehension or disciplinary knowledge. One more thing: GPT-3 can also translate text between languages.
This redditor provided comically specific instructions: “Write me a poem about a war in 2045 between Amazon and Alibaba, in two parts, A for Amazon and B for Alibaba, each written from the perspective of the corporation itself as if it was sentient. And make sure part B is in Chinese.” Another successful output (you can read the whole poem in the link), although GPT-3 seems to struggle with rhyming.
Francis Jervis inputted phrases in everyday English and tasked GPT-3 with adapting the text into legal jargon. For example, “My landlord did not return my security deposit in the time allowed by California law” was transformed into “Defendant has failed to refund to Plaintiff all sums of money paid to Defendant as security deposit, within the time periods specified in California Civil Code section 1950.5.” Jervis did NOT provide the relevant statutes or legal terms; GPT-3 autonomously researched and included that information.
Gwern Branwen used GPT-3 to generate an extensive collection of creative fiction, spanning poetry, science fiction, dad jokes, and more. Branwen glowed about the quality of the work:
GPT-3’s samples are not just close to human level: they are creative, witty, deep, meta, and often beautiful. They demonstrate an ability to handle abstractions, like style parodies, I have not seen in GPT-2 at all. Chatting with GPT-3 feels uncannily like chatting with a human. I was impressed by the results reported in the GPT-3 paper, and after spending a week trying it out, I remain impressed.
(His entire page on GPT-3 is worth reading if you want deeper insights into the tool’s potential — and you really do!)
AI researcher Joscha Bach posted the following tweet about GPT-3:

Jesse Szepieniec did not fully understand the tweet (confession: neither did I), so he inputted the text into GPT-3 and asked the tool to explain the ideas in a full blog post. When Bach read GPT-3’s effort to unpack the ideas in his tweet, he replied, “I am completely floored... it's like 95% meaningful and 90% correct. I don't think that I have seen a human explanation of my more complicated tweets approaching this accuracy :).”
Instead of inputting words, Kaj Sotala tested the tool’s predictive powers for visual information. He submitted partial images and GPT-3 returned (mostly) plausible alternates for the parts that were missing:
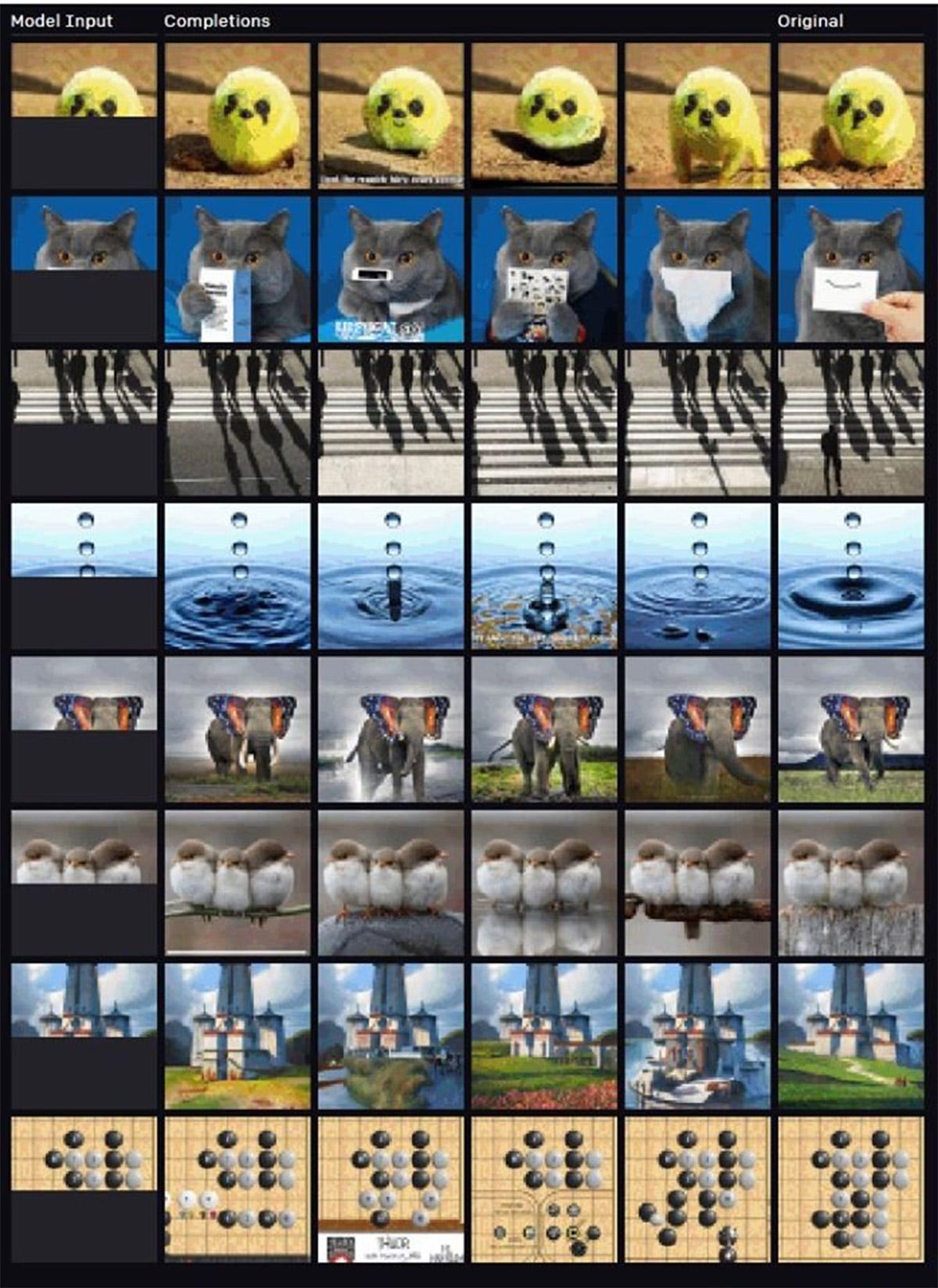
[Edit: Aug 7, 2020- Kaj reached out in the comments below to clarify that the images were NOT his. He pulled them from OpenAI’s blog post, “on the kind of results that they got when they trained GPT-2 on pictures rather than words”]
Mckay Wrigley created an application where you enter the name of a teacher (like “Elon Musk” or “Shakespeare”) and what you want to learn (like “rocket science” or “playwriting”). Once again, GPT-3 was able to draw on massive troves of source text to generate coherent-sounding responses — especially if the teacher was an expert of the topic (i.e., Shakespeare’s advice on playwriting would be more logical than his comments about rocket science). Wrigley’s application was taken down by OpenAI while they “conduct a security review.” AI companies are generally cautious about ways that their technology can be exploited to promote hateful or violent perspectives. In 2016, Microsoft launched Tay, a Twitter chatbot — but then pulled the plug within 24 hours due to misogynistic and racist language.
OthersideAI built a tool where you input your key ideas, and GPT-3 will compose an email in your personal style. Moreover, the tool can take a piece of long-form text and convert it to a bullet point summary.
In just a few hours, Paras Chopra built a fully functional search engine. You enter “any arbitrary query” and “it returns the exact answer AND the corresponding URL.”
Paul Katsen built a GPT-3 tool for Google spreadsheets. If you highlight data in the sheet, GPT-3 can provide information for additional cells. For example, if column A lists the names of a few US states and column B lists their populations, GPT-3 can generate information for all of the other states.
All of these examples demonstrate the incredible potential for GPT-3, and this is from just one week of beta access for a very limited number of users! The tool is incredibly efficient because no coding is required — you just input text and watch with amazement as GPT-3 generates content. And simple “training” — with very few examples — will make the results even better.
GPT-3 Limitations
Although GPT-3 is an astonishing tool, it still has limitations. Keep in mind, the software works by predicting words. And because it generally picks “the most likely next word,” there is an easy way to identify text generated by GPT-3. As Leon Lin explains:
...because of the hyperparameters used in GPT-3, the frequency of words generated will not follow distributions expected from normal humans… this results in common words turning up even more than expected, and uncommon words not turning up at all.
You can fine tune the “temperature” of the algorithm so that it’s more likely to choose uncommon words (effectively giving GPT-3 more “creativity” through randomness). Still, the current iteration of OpenAI’s GPT cannot account for the fact that many humans include rare words at some point in their writing, even though they don’t use those types of words at every point within their writing.
Users can also fool GPT-3 by asking it nonsensical questions — especially ones that have never been asked before in the history of the internet. For instance, the question “How many eyes does a blade of grass have” retuned the answer “one” (instead of the correct answer, zero). But GPT-3 can be trained to answer absurd questions with responses like “that question makes no sense.”
GPT-3 is not “intelligent” in any sense of the word, but it is a little scary what a non-intelligent, non-self-aware tool can come up with. In this tweet, KaryoKleptid shares a simulation that taught GPT-3 math skills by getting it to “show the work” — the same way you would guide a child to learn functions.
GPT-3 and me
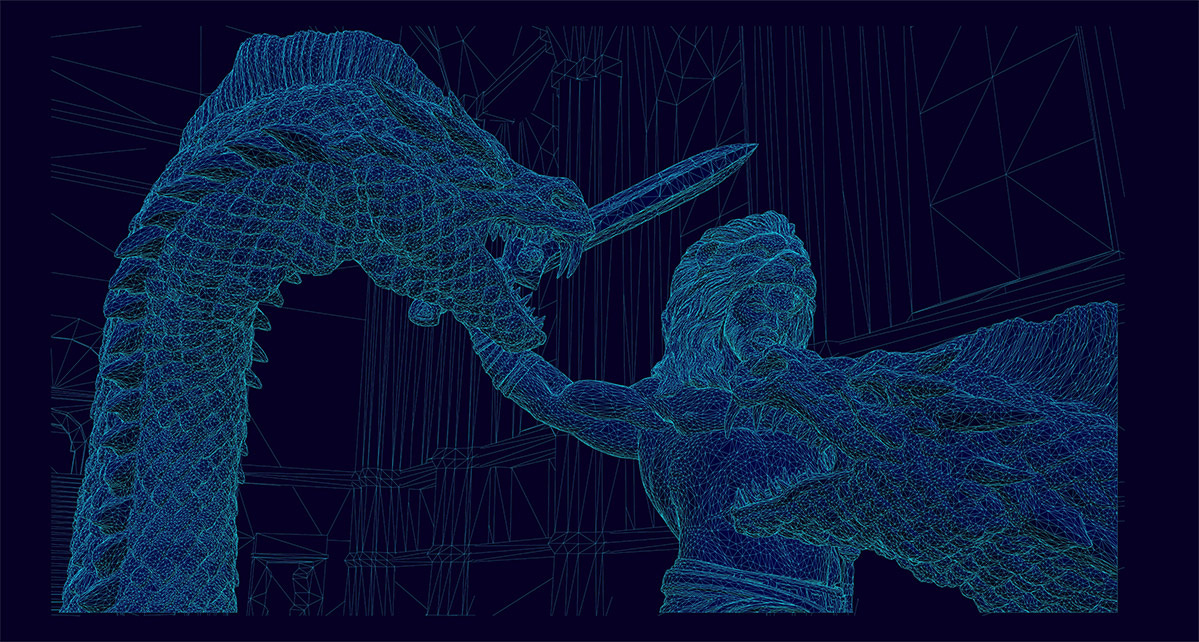
At this point, OpenAI has only provided beta access to GPT-3 for a limited number of users. You can apply for access, which requires a brief proposal about your plans (beyond just a desire to play!). If you aren’t lucky enough to gain access, you can’t even “borrow” someone else’s because the terms of use prohibit sharing (plus, you need to log in with your personal Google account).
But… Nick Walton created a free-to-play game that uses GPT-2 (OpenAI’s 2019 version). AI Dragon is a text-based roleplaying game (that you simply MUST try — even if you have no experience or interest in roleplaying games). Over the last 30 years, there have been many roleplaying video games, but this one uses GPT as the “Dungeon Master,” leading to limitless options. On July 11, Walton received access to GPT-3. As a new option, you can “upgrade your AI Dungeon experience to the more advanced AI for $9/month” (called “Dragon Mode”).
The latest version of AI Dungeon allows users to create custom scenarios. With this option, you can effectively access a (slightly modified version) of GPT-3. Walton’s work was designed as a game, so as long as you “lean in” to some basic constraints, you can experiment with a product like GPT-3 for a reasonable fee.
I used the tool create Twilight Zone episode summaries, tweets in the style of Naval Ravikant and Donald Trump, and fictional Malcolm Gladwell book descriptions.
Plus, I developed a simulation where you play as a CMO working with a consultant to solve business challenges. You can play the scenario here (note: unless you sign up for AI Dungeon’s “Dragon Mode,” you will play my scenario with GPT-2).
Getting the tool to produce the desired results takes some work. I expect that many of the “amazing” examples I shared above took people more than one attempt to perfect. In many cases, when people try to replicate someone else’s output, they end up with something much less impressive. Small changes in inputs, or different “temperature settings,” can lead to vastly different results.
After playing with my CMO scenario a few times, I built a version where the consultant is in the room with you, ready to help with the specific goal of developing a brand name for your company. With the more detailed premise, the game was vastly better. You can read the full conversation where I collaborated with the consultant to name a new brand of water bottle (we settled on “Surge”). I even managed to have the program write me a jingle for the product:
The consultant spends a month writing a new jingle for the water bottle and then reports back ready to sing the song. She warms up her voice, and then begins to sing the jingle:
“Grab your bottle, grab your cap!
It’s time to go!
A journey of a thousand drops begins with M-M-M-M-M,
SURGE!
Will West (a Marketing BS reader who also follows me on Medium) came across my CMO simulation. He used the idea to build a version for financial planning. You can watch his video here.
The Future
OpenAI estimates that training a new GPT-3 with specific parameters would cost approximately $4.6 million. After the beta period, API access will cost “something” (the company is currently determining its pricing models). The cost structure will obviously impact who can use the tool and in what capacity. While the vast majority of companies lack the resources to create their own GPT, I expect the big tech players (Amazon, Google, Apple, Facebook) will consider the idea. I know how much I would appreciate a more intelligent Echo and Siri.
Once GPT-3 is out in the wild, what will it mean?
I do NOT think GPT-3 will cause a massive elimination of jobs. Yes, the tool will allow companies to create SEO content at scale with minimal human involvement. But just as the introduction of ATMs actually INCREASED the number of bank tellers, I expect that GPT-3 could boost the number of content jobs — with a different focus. Instead of creating all the content themselves, these people will determine the best prompts, parameters, and structures to optimize the output of GPT-3.
For instance, SloppyJoe API (a service that creates digital handwriting) developed a tool that gives a writer ways to change the style or tone of their work:

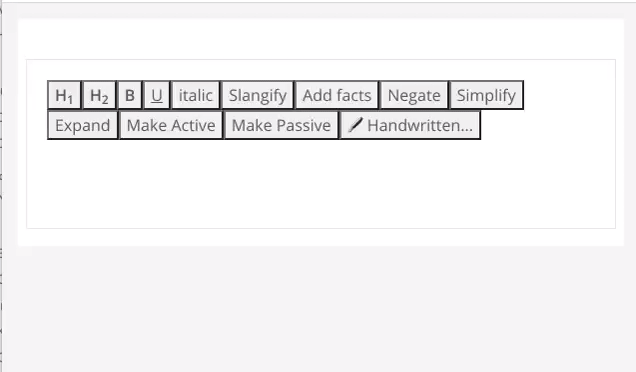
For some context about the evolution of artificial intelligence, think about chess. When the first chess-playing computers were introduced, they were barely competent (they understood how the pieces moved, but not much more). Over time, the programs were about as good as amateur chess players. Eventually they surpassed the skills of the best chess players in the world. But even then, teams of chess players with access to computers (aka “freestyle chess” or “centaurs”) could consistently beat computers alone (or humans alone).
Today, GPT-3 is not as good at writing as the best authors, but the tool’s literary proficiency IS about as good as many amateur writers. I am not sure we have reached the stage where a team of humans and computers could rival or outshine the most talented human writers, but it might not be long.
Last month, after COVID had spread over the planet and Black Lives Matter protests swept across America, I asked my wife, “What is the probability that the most surprising event of 2020 has not happened yet?” We both laughed. Of course, nothing could be bigger than what already happened.
We might have been wrong.
Unless there is another big surprise, I expect to explore AI’s impact on marketing in next week’s letter.
Keep it simple, stay safe, and play with AI Dungeon Dragon Mode,
Edward
If you are enjoying Marketing BS, the best thing you can do is share it with friends and recommend they subscribe. The next best thing you can do is comment on this piece or click the heart (which makes this article more visible for other readers).
Edward Nevraumont is a Senior Advisor with Warburg Pincus. The former CMO of General Assembly and A Place for Mom, Edward previously worked at Expedia and McKinsey & Company. For more information, including details about his latest book, check out Marketing BS.
> Instead of inputting words, Kaj Sotala tested the tool’s predictive powers for visual information. He submitted partial images and GPT-3 returned (mostly) plausible alternates for the parts that were missing:
Actually, it wasn't me who sent those pictures to GPT-3; rather those pictures were from OpenAI's blog post on the kinds of results that they got when they trained GPT-2 on pictures rather than words.